- Jan. 20, 2022
- 7 min
Surface After a Deep-Dive (Zen and the Art of Analytics)
Magnolia in Aktion
Unser Expertenteam zeigt Ihnen live, was Magnolia für Sie leisten kann.
Jetzt Demo buchenWhen you’re working in DX, you have a lot of data on your users. So much data that it becomes hard to figure out what to do with it. How do you avoid data overload paralysis and still come up with actionable data instead?
There’s a multicolored spectrum ranging from going with gut instinct on one end to over-researching and getting stuck in a deep dive in complexities on the other. Given the amount of data available to us in digital experience, it’s easy to take deep dives. But if you don’t resurface with actionable insights, you’ll drown in the data lake.
Overdoing the Research
A friend of mine did a PhD in Law and then started working as a lawyer. I asked him how the transition was going. He laughed. “Well, one of our clients is in ecommerce, so I was asked to write a memo about the EU e-Commerce Directive. I worked on it for about a week and sent it to the partner I work for.” The feedback he got on it was that it was a great piece of research. However, the client had a very specific question. Are we allowed to do this? It didn’t need a twenty-page memo on the nuances of the law. It needed an actionable “yes, and this is how.”
I was working on a recommendations engine; we had a data lake with dozens of different sources, which could give us – I thought – the combination of all angles on any user to infer the perfect next video to play. The lead architect tried various approaches and a bunch of test cases to determine the best algorithm to use. We had a call about the results of his research, and I asked him about his conclusions and what data he would advise us to use. There was an almost embarrassed pause, and he said, “As it turns out, the only thing that matters is which videos a user played before, and for how long.” And I laughed. It was much easier and cheaper to run it that way! Sometimes you don’t need all the data in the world; you only need the relevant data, but figuring that out can be a lot of work.
Genius Design
Steve Jobs is, famously, associated with “genius design”. User research wouldn’t have given us the Mac, the iPod, or the iPhone: it took great new ideas, then polished to perfection.
It’s very appealing, and for good reason. You can’t ask your users directly what they’d want – they’re not the experts. They expect you to deliver the experience to them. Trying to elicit the answers from the data can be equally difficult. It may seem better to “think for your user”, put yourself in their shoes and then come up with what they need. That’s valuable, of course: never forget the audience! But it’s easy to get off track and then completely diverge from what the actual audience thinks about it. Not in the least because quite often, you won’t be typical of your audience yourself.
Before you say, “I know what our audience wants,” ask yourself; are you Steve Jobs? If not, be very careful with genius design.
The Scientific Method
In science, you’d start with one (or several) good questions. That’s followed by exploratory research, which eventually leads to a hypothesis. You then have something you can prove or disprove with data.
In DX, you can similarly come up with questions and hypotheses, and there are a plethora of tools to help you judge the results. It’s easy to run A/B tests on emails and notifications. If you’ve spent the time to properly integrate it into the foundation of your platform, running multivariate tests on screens shouldn’t be a problem. And you can dig into your data lake and see if you can connect the dots on many other questions.
Of course, one of the first things you discover is that it’s nowhere near as accurate or clear-cut as you’d expect from digital data points measured in real-time. The discrepancy between data silos can be stunning. I’ve routinely seen a 15-20% difference between analytics tools purporting to measure the exact same thing. Or between server log analysis and end-user analytics.
There are many reasons for those discrepancies – for one, have a very careful look at how each tool or data set defines the criteria. And while digital is supposed to be about very black and white, ones and zeros, data does get lost, both in receiving the pings and processing the aggregates. You have to be aware of this, and you should invest time in understanding the differences. But it can still feel like guesswork, decidedly un-scientific and messy.
And that’s fine. It reminds me of one of my favorite “Far Side” cartoons. It shows a crooked rocket, misaligned between the stages, with the caption, “It’s time we face reality, my friends… We’re not exactly rocket scientists.” And that’s exactly it. Digital experience is difficult, but it’s not exactly rocket science. If you get your hypothesis wrong, you can correct it in the next sprint. You won’t have to deal with the fallout of an exploding satellite.
Over the holidays at the end of last year, the James Webb Telescope was launched and deployed. Getting it right meant passing 344 potential single points of failure. After thirty years of research and design, they actually managed to pull that off. And I also sighed a breath of relief; in DX, not only do you get to engineer around SPOFs; but if you do hit one, there is always a workaround, and you can always go back to the drawing board and try again.
In digital experience, use the scientific method, form your hypothesis, and test it against the data – but don’t overdo it. Launching is more important than avoiding any risk. And we’re not exactly rocket scientists.
The Intuitive Method
As a kid, before the internet, I’d read anything I could get my hands on. That’s how I ended up reading “The Inner Game of Tennis”, even though I didn’t play tennis, it stuck in my mind. One example is that you shouldn’t think while trying to hit the ball. You can think through the movement before you play, but while you’re swinging the racket, you shouldn’t be thinking. The book is more about Zen than it is about tennis. You might as well apply it to data, analytics, and research.
Years ago, we were trying to come up with a new product. My suggestion was to mock up interactive wireframes, and test those with ad hoc focus groups – unscientifically, if need be just using our colleagues from other departments. We could then create an MVP from that and test that again. Once we launched that, we could add features through constant multivariate testing.
Of course, as always, the launch was up against an aggressive deadline, and there was no time for any of that. But while we managed to launch the service relatively quickly by skipping the research, it got killed two years later – when we had to acknowledge it didn’t resonate with the target audience. It was genius design gone wrong. Hitting the ball hard but in the wrong direction.
However, the good news is this doesn’t mean that intuition is not a thing. But in digital experience, you first need to feed that intuition before you can trust it. If you’ve gone through enough cycles of questioning and then proving or disproving hypotheses with data, you can start skipping steps selectively more and more because you’ll actually know what the result would be (instead of just wishing it). And once you get in the evolutionary stage, this can start feeding constant feedback loops, leading back to questions that produce hypotheses.
You could call it “The Inner Game of Data”, but I prefer “Zen and the Art of Analytics”. Research, launch, review. Don’t get stuck in the research phase – but that doesn’t mean you should just whack about aimlessly.
Read more from Adriaan Bloem
This guest blog post is the last of a series of five articles, written for Magnolia by Adriaan Bloem:
Oh, and a sweet surprise for you: Adriaan is not only writing blog articles for you, he also wrote a book:
Audio Blog
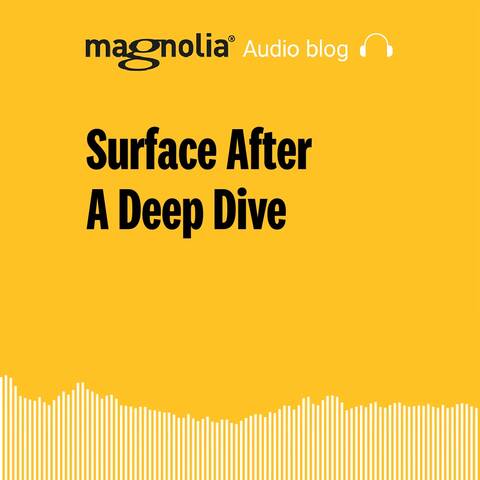